News Story
Evaluating the Use of LLM Agents to Provide Better Software Security
Published November 8, 2024
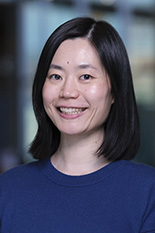
As large language models (LLMs) continue to take on complex tasks previously done by humans—analyzing RNA for vaccines, writing software code, generating news articles, and much more—how does the technology fare at preventing cyberattacks on critical infrastructures like financial institutions or energy grids?
LLMs are already being used to launch cyberattacks, with cybercriminals exploiting malicious inputs to LLMs to generate malware, create phishing emails and phishing sites.
Other AI-infused technologies, known as LLM agents, are even more powerful than just LLMs alone, with the large language model serving as the core language engine, and additional computational enhancements making the “agent” more powerful and versatile.
Security experts are just now starting to use LLM agents to counter cyberthreats. They would benefit immensely from a comprehensive set of benchmarks that can validate the technology’s efficacy at any level, whether it’s protecting your laptop from teen hackers or safeguarding financial systems and public utilities from foreign adversaries.
A University of Maryland cybersecurity expert is hoping to give these validation efforts a boost, working to develop end-to-end benchmarks as well as developing state-of-the-art LLM agents to perform a complete cyberdefense workflow process—from vulnerability detection, to analysis, to software patching.
Assistant Professor of Computer Science Yizheng Chen is principal investigator of the two-year project, funded by a $1.7 million award from Open Philanthropy, a grantmaking organization that aims to use its resources to help others.
LLM agents are like the robots inside the computer, Chen says. They can use software tools, take actions, self-reflect, interact with the environment, and maintain long-term memory. They are designed to exhibit more autonomous and goal-oriented behavior, providing greater cyberdefense capabilities.
The key to her project, Chen explains, is to build very difficult cybersecurity benchmarks that the LLMs have not been trained on, avoiding the possibility of LLM agents memorizing the solutions.
“We are looking to find some of the most challenging software vulnerabilities to begin building the benchmarks on. This allows us to use ‘human-discovered solutions’ as our ground truth,” says Chen, who has an appointment in the University of Maryland Institute for Advanced Computer Studies and is a core faculty member in the Maryland Cybersecurity Center.
The project will also involve multiple LLM agents that Chen is developing with cyberdefense techniques. The rapidly advancing outputs of LLM agents can ensure that any AI-generated solutions meet rigorous standards in three areas, she says. This includes a) that any software vulnerability is found; b) that the vulnerability is properly analyzed; and c) that any vulnerability patches satisfy both functional correctness and security requirements.
To help in this area, Chen says that she anticipates using some of the research funding to bring on several new Ph.D. students, either recruiting fresh talent from outside the university or tapping into the rich pool of talent already at UMD.
“I am grateful that there is already a very strong cybersecurity and machine learning community here at Maryland—both in our faculty colleagues, current students and postdocs, or the excellent support staff we have,” she says.
Ultimately, Chen believes that AI-generated cybersecurity solutions will be the standard moving forward. But for that to happen, there must first be a high level of confidence that computer-generated cyber fixes are efficient, effective and trustworthy.
“I am deeply thankful to the support from Open Philanthropy. I hope this project moves the science forward significantly,” she says.
—Story by UMIACS communications group